How Much Does AI Development Cost in 2025? A Complete Breakdown
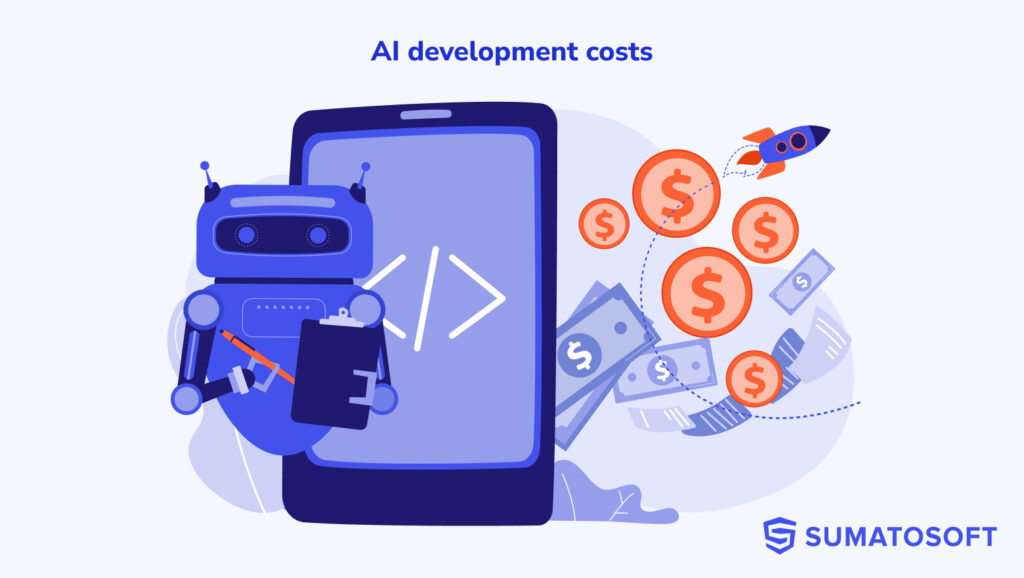
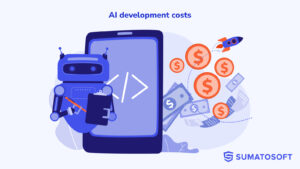
A decade ago, Artificial Intelligence was a futuristic concept that book authors wrote about. Actually, AI wasn’t a very popular book topic.
Nowadays, AI is a strategic necessity for businesses in every industry, thanks to its ability to be integrated into almost any business process – be it customer service, supply chain management, stock tracking, fraud prevention, machinery maintenance, and more. AI’s impact is growing, and it is here to stay.
The adoption is accelerating, but what about the required investments? AI remains a black box for many decision-makers because its opportunities seem almost unlimited, while the required budget for implementation remains unclear. In 2025 and beyond, understanding the cost of developing and implementing AI is no longer optional. It’s a key part of business planning, risk management, and ROI forecasting.
This article offers our accumulated knowledge on the costs of building AI solutions. We at SumatoSoft have developed multiple custom Artificial Intelligence solutions and provided OpenAI development services to businesses across 27 countries. This article reflects what we’ve learned from this.
AI Development Cost at a Glance
First, let’s briefly answer one question: how much does AI cost?
Just as with other software solutions, multiple factors affect the overall development costs of AI solutions. However, there is a superior factor: project complexity, which splits all software solutions, including one with AI, into 4 groups.
Complexity level | Example projects | Estimated cost range (USD) | Development time |
---|---|---|---|
Simple | Rule-based chatbot, form auto-fill, AI FAQ assistant | $5,000 – $50,000 | 1–2 months |
Moderate | ML-based recommendation engine, customer sentiment analysis | $50,000 – $150,000 | 2–4 months |
Advanced | Computer vision, predictive maintenance, NLP-driven assistant | $150,000 – $400,000 | 4–6 months |
Enterprise/Complex | LLM-powered app, real-time AI for fintech or healthcare | $400,000 – $1,000,000+ | 6–12+ months |
AI Development Cost Factors Breakdown
So, project complexity is the primary AI cost driver. Let’s review the others:
- development model (in-house, outsourced, hybrid);
- AI type (rule-based, ML, DL, LLMs, NLP, computer vision);
- project complexity and goals;
- data: quantity, quality, labeling, privacy compliance;
- talent: team composition & rates;
- infrastructure: cloud vs on-premises vs hybrid;
- third-party APIs and model usage (e.g., GPT-4, Claude).
Here is a brief factor overview:
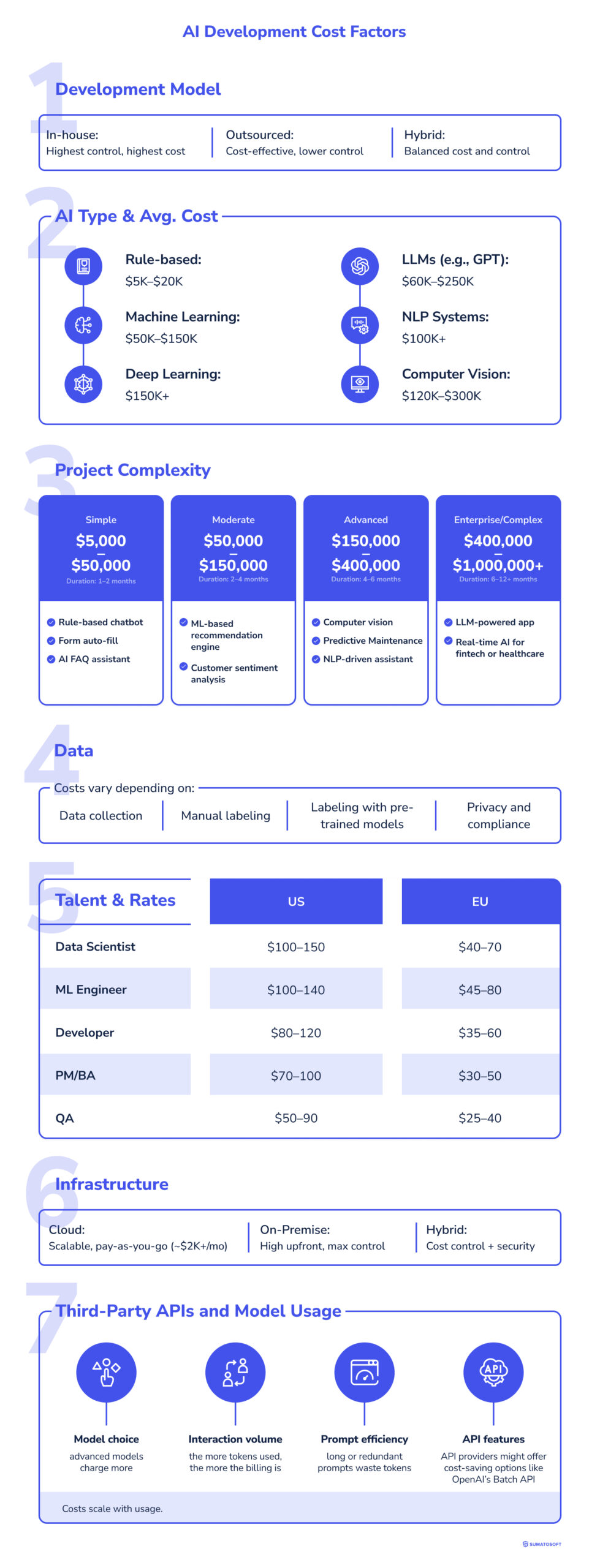
Now we’ll break each of these AI development cost factors down in more detail.
Development Model
- In-house – the company hires specialists in-house, managing them and handling all aspects of development and operations. This model offers the highest control, but is also the most expensive.
- Outsourced – the business partners with an external software development provider and fully outsources the process of AI development and implementation. This model is cost-efficient and fast to start, but lacks control over the flow and implies risks of finding an unreliable vendor.
- Hybrid – this one is the mix of the previous two models. The company keeps key roles in-house, usually a project manager or tech lead, and collaborates with an external team for development and support. This model combines the control benefits of in-house development and the cost-efficiency of the outsourcing model.
Developer’s comment: Hybrid models are growing popular.
AI Type
AI type is a part of the complexity factor because it defines the type of technology involved in the development. Below is a breakdown of different AI types:
- Rule-based systems – used for simple, predictable tasks like basic chatbots or automated responses with predefined rules. Ideal for low-budget projects with limited complexity.
- Machine learning – used for tasks requiring pattern recognition, predictions, or classifications (e.g., recommendation systems, fraud detection) where a vast volume of data is available and doesn’t require deep analysis.
- Deep learning – used for complex tasks with large datasets, like image recognition, speech processing, or autonomous systems.
- LLMs (e.g., GPT, Claude) – used for natural conversations, content generation, and text analysis that requires contextual understanding.
- NLP systems (summarization, chat) – used for specialized language tasks like summarization or sentiment analysis.
- Computer vision – used for image or video analysis tasks (e.g., facial recognition and object detection) that require heavy data processing and GPU resources.
AI type used | Weighted average development cost |
---|---|
Rule-based systems | $5K–$20K |
Machine learning (ML) | $50K–$150K |
Deep learning (DL) | $150K+ |
LLMs | $60K–$250K for MVPs |
NLP systems | $100K+ |
Computer vision | $120K–$300K |
We can highlight the simple dependence here:
More advanced AI types require more computable power, data volume, and more robust infrastructure, which raises the cost.
Project Complexity and Goals
We’ve already discussed project complexity as the primary driver of AI development costs. Here is a brief summary:
- we can categorize all projects into simple, moderate, advanced, and enterprise levels of complexity;
- project complexity is determined by the scope, technical requirements, custom pipelines, processing requirements, infrastructure needed, integration requirements, and AI type required.
The more complex the AI logic, data flow, and expected accuracy, the higher the AI development cost.
Data: Quantity, Quality, Labeling, Privacy Compliance
Data is the lifeblood of AI, so it’s necessary to set proper governance practices and follow privacy compliance requirements. Below, there is a breakdown of factors that define AI costs:
- Data collection – can be nearly free for existing internal data or expensive if it requires integration with external datasets like Global Health Data Exchange, Bloomberg’s financial data, or NASA’s satellite imagery.
- Manual labeling – manual labeling for complex tasks like annotating medical images. It’s a labor-intensive task and can cost $30K or more.
- Labeling with pre-trained models – an alternative to manual labeling is using of pre-trained models, which still require fine-tuning with task-specific data to achieve optimal performance.
- Privacy and compliance – security measures like encryption and anonymization add to the budget, especially in healthcare or finance.
Talent: Team Composition & Rates
Where you hire makes a significant cost difference – outsourcing to skilled vendors in Eastern Europe or LATAM can cut AI costs by up to 60%.
Role | US Rates ($/hr) | Eastern Europe Rates ($/hr) |
---|---|---|
Data Scientist | $100 – $150 | $40 – $70 |
ML Engineer | $100 – $140 | $45 – $80 |
Backend Developer | $80 – $120 | $35 – $60 |
PM / BA | $70 – $100 | $30 – $50 |
QA Engineer | $50 – $90 | $25 – $40 |
Infrastructure: Cloud vs On-Premises vs Hybrid
The infrastructure choice depends on the project needs and requirements, so it’s not something that should be done immediately. The service vendor selects the most suitable options after the initial discovery phase, when the requirements are clarified. Here is a brief summary of the available infrastructure options:
- Cloud (AWS, GCP, Azure) – all data is stored on cloud servers, a pay-as-you-go billing method where you need to pay only for used capacity, highly scalable due to the cloud nature, and fast to deploy. Suitable for most use cases.
- On-premise – all data is stored on local servers owned and maintained by the organization, requiring high upfront costs for hardware, setup, and maintenance. It is highly secure due to full control over data and infrastructure. Suitable for data-sensitive projects or massive workloads.
- Hybrid: Often used to control costs – data is processed on-prem, AI is trained in the cloud. – combines local and cloud servers, where sensitive data is stored on-premises while AI models are trained and deployed in the cloud. This is an intermediate option that offers a good balance between security and AI cost.
Infrastructure costs often start at ~$2,000/month for cloud-based AI workloads and scale fast with compute usage.
Third-Party APIs and Model Usage (e.g., GPT-4, Claude)
Most third-party AI model APIs charge based on tokens, which are units of text processed by the model. A token is 4 characters or 0.75 words in English. AI pricing is split into input tokens and output tokens, which are user prompts and AI model responses, respectively.
Most third-party APIs’ pricing model is pay-as-you-go, with no upfront costs for infrastructure or servers. We examined pricing models in our article, the best 10 ChatGPT alternatives. However, training an accustomed model is a different topic with different pricing.
Factors that drive costs are:
- Model choice – advanced models charge more.
- Interaction volume – the more tokens used, the more the billing is.
- Prompt efficiency – long or redundant prompts waste tokens.
- API features – API providers might offer cost-saving options like OpenAI’s Batch API.
Costs scale with usage. A chatbot handling millions of tokens monthly (e.g., 20M input + 10M output) could cost $500–$5,000+ with GPT-4 Turbo a month. So, check the AI pricing first.
Cost Samples for Several Common AI Project Types
AI can be embedded literally in any field, process, or industry. The issue here is why AI should be embedded rather than where and how. So, the list of AI project types would be infinite, if not limited.
We decided to gather several of the most popular AI project types that we dealt with.
Use Case | What’s included | Cost | Timeline | Key cost drivers |
---|---|---|---|---|
1. AI chat assistant (MVP) | Basic chatbot, answering FAQs, customer support, CRM integration, and usage of pre-trained NLP models. | $5,000 – $50,000 | 1–2 months | Number of supported integrations, languages, and overall UI complexity |
2. Generative AI MVP | Lightweight app using LLMs (e.g., GPT, Claude) to generate content, text, images, or code, and API integration. | $60,000 – $150,000 | 2–3 months | LLM usage fees, API volume, and number of integrations |
3. Predictive analytics dashboard | Data ingestion, custom ML model training, charts and alerts, admin module, data export features, basic forecasting engine | $80,000 – $200,000 | 3–5 months | Data quality, complexity of tracked metrics, forecasting complexity, infrastructure (cloud or on-prem), model retraining |
4. Real-time computer vision system | Image/video input processing, object detection, edge/cloud inference pipeline | $120,000 – $300,000 | 4–6 months | Frame rate, number of data sources, model size, GPU needs, data labeling, and on-device deployment requirements |
5. Voice/NLP assistant | Voice input support, transcription, NLP parsing, response engine, multi-language support, API integration | $100,000 – $250,000 | 4–6 months | Speech-to-text accuracy, model training, noise handling, languages supported, integration with phone or app platforms |
6. AI scoring & risk evaluation tool | Risk model (e.g., credit scoring, fraud detection), data preprocessing, scoring engine, result dashboard UI, compliance requirements | $120,000 – $300,000 | 5–7 months | Regulatory compliance, data processing requirements, the depth of model solutions’ explainability,the number of incoming choice criteria, and retraining |
Industry-Specific AI Development Cost
Different industries adopt AI in unique ways, shaped by their specific needs, regulations, and data environments. The AI app development cost and complexity of AI projects vary widely by sector. We gathered general use cases across several industries with estimated budgets and timelines.
Industry | Example use cases | Cost | Timeline |
---|---|---|---|
Finance | Fraud detection, risk scoring, AI-based loan processing | $100,000 – $400,000 | 4–6 months |
Healthcare | Medical imaging (CV), patient triage assistant, EHR summarization (NLP) | $150,000 – $500,000+ | 5–8 months |
Retail & eCommerce | Personalized product recommendations, demand forecasting, AI customer support | $80,000 – $300,000 | 3–6 months |
Logistics | Predictive maintenance, route optimization, inventory demand planning | $120,000 – $350,000 | 4–7 months |
Manufacturing | Quality inspection with computer vision, process automation, preventive maintenance, equipment failure alerts | $100,000 – $400,000 | 4–7 months |
Hidden and Ongoing Costs You Shouldn’t Write Off
We have examined the costs associated with developing an AI solution. However, building an AI solution is only part of the financial picture. Let’s shed some light on additional AI development costs that should be considered when assessing the total cost of ownership of an AI solution. There are 5 cost types associated with ongoing activities after the project launch.
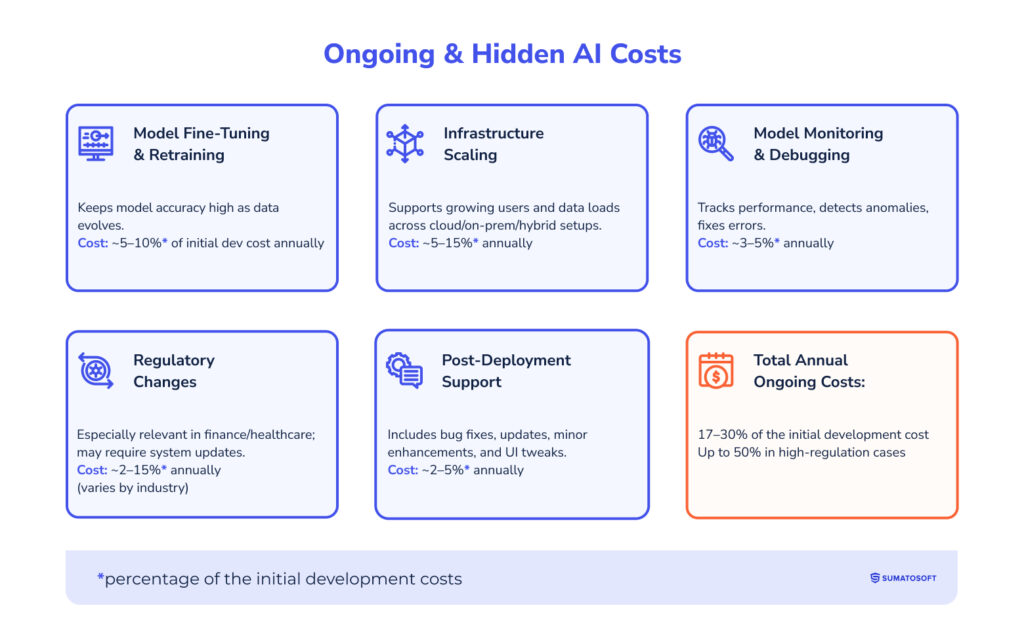
#1: Model Fine-Tuning & Retraining
AI models degrade over time as data changes. Regular fine-tuning or retraining is needed to maintain accuracy, especially for customer behavior, fraud, or operational models. A good practice here is to run major retraining and fine-tuning 1-2 times a year.
Estimated costs: ~5–10% of initial dev cost annually.
#2: Infrastructure Scaling
As we’ve already mentioned, the growing number of users implies the need for growing infrastructure, compute capabilities, storage, GPU power, etc. This cost applies to all infrastructure types: cloud, on-premise, and hybrid.
Estimated costs: ~5–15% of initial dev cost annually depending on volume and infrastructure type.
#3: AI Model Monitoring & Debugging
This AI development cost aspect is unique to AI systems, and it’s about monitoring the AI model’s behaviour and health. AI developers track model accuracy, latency, and performance, detecting drift of model degradation, alerting when behaviour anomalies happen, debugging misclassifications and hallucinations, and logging performance metrics.
Estimated costs: ~3–5% of initial dev cost annually (for tools + minimal engineering time)
#4 Regulatory Changes
(especially in healthcare/finance)
This additional aspect is especially acute for highly regulated industries like healthcare or finance. Compliance updates may require retraining the model or rebuilding parts of the system. These costs can be unpredictable and significant.
Estimated costs: ~2–15% of the initial dev cost a year when regulatory requirements change. The exact cost depends on the industry and regulatory impact.
#5 Post-Deployment Support & Updates
These are usually maintenance costs that are relevant to all software solutions. They include bug fixes not related to the AI model, performance optimizations, technology updates, security patches, minor feature enchancements, and UI tweaks after the launch.
Estimated costs: 2–5% of initial dev cost annually
Total Ongoing and Hidden Costs Estimate
Likely around 17–30% of your initial AI development cost per year, with up to 50% in the worst-case scenario. Regulatory changes are the most unpredictable, but still should be considered. The latest report on the AI index from Stanford University identified that the number of AI-related regulations in the United States doubled in 2024 compared to 2023.
AI Development Cost Without a Clear Purpose and Goal
We have repeatedly received leads with brilliant ideas for using AI that ultimately never reached the development stage. The reason is simple: these ideas lacked an understanding of why AI was needed and what it would achieve.
This issue isn’t limited to startups with innovative ideas. Businesses approach us with requests like “We want to integrate AI into our business to increase efficiency,” without specifying what efficiency means, where AI should be applied, or why they concluded that AI is necessary.
We acknowledge that AI has the potential to improve business processes in virtually any industry. However, it’s critical to understand which processes and why.
To address this, we offer a free project evaluation, which includes a high-level Discovery Session to identify opportunities for AI implementation and get AI pricing. Read about our approach to project estimation in software development in our article.
We also provide AI development and consulting services, where we thoroughly analyze the business, pinpoint areas for AI integration, select the most suitable technologies, develop an implementation strategy, design the solution architecture, and help form a comprehensive vision for AI within the company’s operations.
All you need to do is contact us.
To answer the question of this section, we can say that the ROI from implementing AI without a clear purpose often approaches zero, and the investment volume is ongoing because all solutions seem inadequate.
How to Justify the AI Development
A lot has been said for now, but let’s dive a little deeper. This section will be relevant for startups planning to pitch an AI project and businesses considering implementing AI and experiencing difficulties in securing budget approval from stakeholders.
Below are practical ways to frame and justify AI development costs.
5 Proven Evaluation Methods for AI Projects
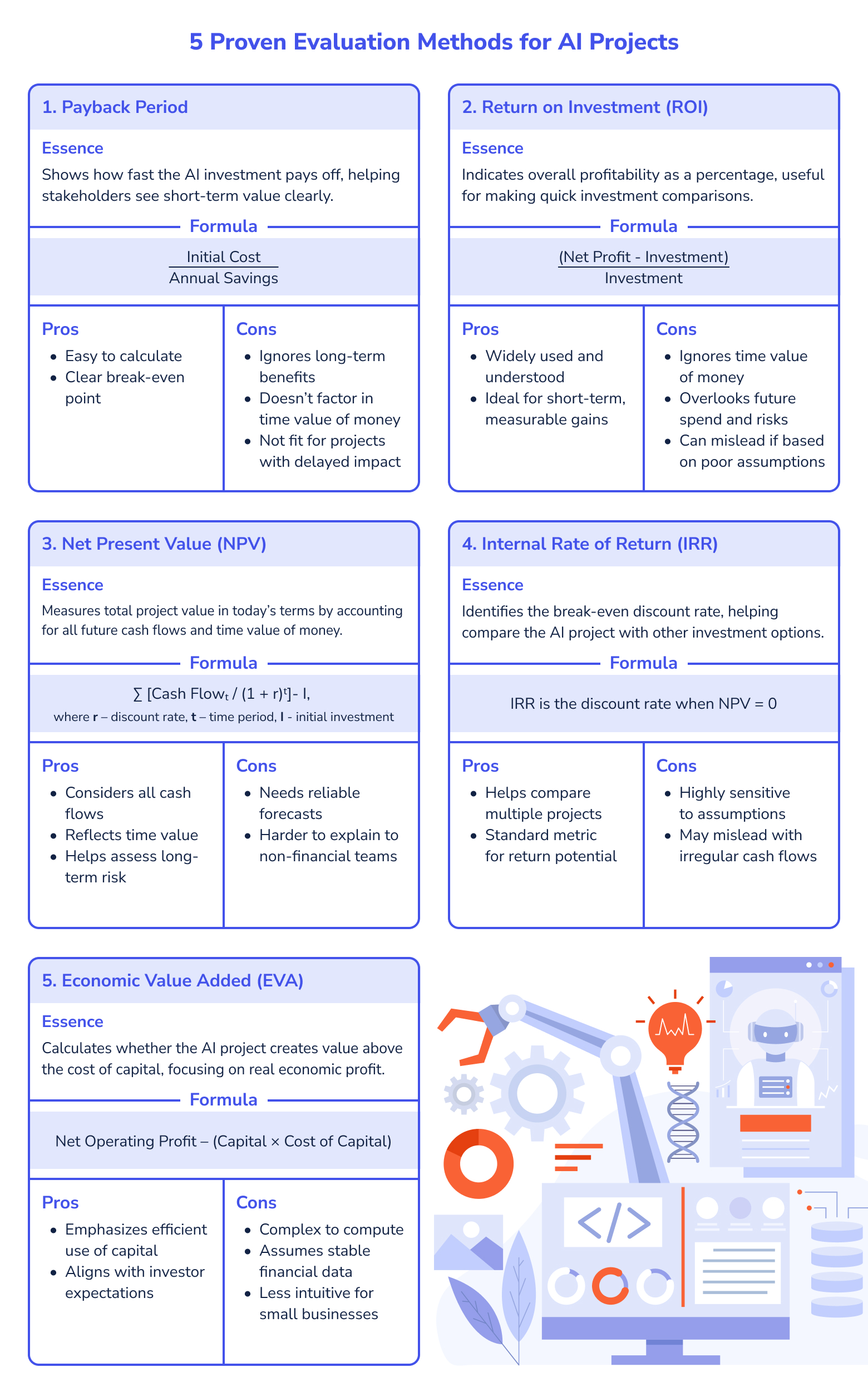
These methods demonstrated great benefits for the AI projects we at SumatoSoft developed. They are:
- payback period;
- return on investments (ROI);
- net present value (NPV);
- internal rate of return (IRR);
- economic value added.
Let’s examine them from several perspectives: the essence, formula, benefits, and weaknesses.
Payback Period
The essence: it measures the time (in years or months) to recover the initial investment through annual savings. Where annual savings is the amount of money the business saves each year by implementing AI.
Formula: Initial Cost / Annual Savings
Benefits:
- simple to calculate and explain;
- shows how quickly the investment is recovered.
Weaknesses:
- ignores profits after payback;
- doesn’t account for the time value of money;
- not suitable for long-term AI projects with delayed impact.
Return on Investment (ROI)
The essence: Measures total financial return as a percentage of the investment. Allows for capturing the overall expected profitability from AI implementation.
Formula: (Net Profit – Investment) / Investment
Benefits:
- widely understood and used;
- works well for short-term or cost-saving AI use cases with immediate, easily measurable financial impacts.
Weaknesses:
- ignores the time value of money
- oversimplifies complex outcomes;
- doesn’t capture risk or future spending on the project, like regulatory change;
- easy to misrepresent with poor assumptions.
Net Present Value (NPV)
The essence: a metric that calculates the current value of all future cash flows from the project, both inflows and outflows, minus initial investments. The metric is discounted to account for the time value of money.
Formula: Σ [Cash Flowₜ / (1 + r)ᵗ] – I, where r – discount rate, t – time period, I – initial investment
Benefits:
- factors in the time value of money;
- takes into account all cash flows over time;
- supports risk assessment;
- is suitable for long-term, multi-year AI investments.
Weaknesses:
- requires accurate forecasting of future returns;
- can be difficult for non-financial stakeholders to grasp.
Internal Rate of Return (IRR)
The essence: is the discount rate that makes the net present value of a project’s cash flows equal to zero. IRR serves as a break-even point. By comparing IRR with the cost of capital it’s possible to understand if the project is profitable.
Formula: IRR is found by solving NPV = 0
Benefits:
- helps compare AI against other investment opportunities based on the return potential;
- standardized familiar metric (percentage);
- useful for prioritizing between multiple projects.
Weaknesses:
- highly sensitive to input assumptions;
- may produce misleading results if cash flows are irregular.
Economic Value Added (EVA)
The essence: it measures the economic profit of an AI project the exceed the minimum required by investors. Unlike ROI which focuses on raw profitability, EVA accounts for the opportunity cost of capital, allowing to assess whether the project worths resources.
Formula: Net Operating Profit – (Capital × Cost of Capital)
Benefits:
- focuses on real value created beyond the cost of capital;
- encourages efficient capital use;
- helps justify AI investments to finance and leadership.
Weaknesses:
- complex to calculate;
- assumes stable financial metrics;
- less intuitive compared to other metrics;
- best suited for large enterprises with defined capital structures.
Non-Financial Evaluation Metrics: Still Powerful
AI doesn’t always show immediate financial gain, so it’s possible to use alternative non-financial evaluation metrics. They can’t solely justify the investments in AI unless transformed into financial metrics (for example, employee productivity in human hours saved) and should be used in combination with financial metrics. Below is the list:
- speed-to-market;
- employee productivity;
- customer experience;
- brand positioning.
Tools For Measuring Financial Gain From AI Projects
Many platforms offer tools that help with Artificial Intelligence cost estimation. Some of them even developed an ROI calculator to simplify this process. Still, the financial gain could be easily calculated independently with the help of the following tools:
Tool Name | Key features | How to use the tool |
---|---|---|
Aible | ROI-optimized AutoML blueprints continuous monitoring what-If analysis integration with business applications | Aible provides built-in ROI optimization features. It guides users through defining business-specific cost-benefit tradeoffs and operational constraints, then automatically generates a portfolio of AI models optimized for maximum ROI. |
DataRobot ROI Calculator | Profit Curve custom evaluation metrics AI Observability payoff matrix configuration business value estimation tools | DataRobot includes tools to calculate and monitor ROI. Its Profit Curve feature allows you to define a payoff matrix that assigns monetary values to true positives, false positives, true negatives, and false negatives. This allows for estimation of the financial impact and determines the optimal classification threshold to maximize profit. Additionally, DataRobot’s AI Observability tools enable tracking of custom ROI metrics over time, providing insights into model performance and business value. |
Google Vertex AI Pipelines | cost tracking via billing export custom labels for pipeline runs performance metrics monitoring integration with BigQuery for analysis | Vertex AI Pipelines can assist in calculating ROI by enabling detailed tracking of pipeline costs and performance metrics. You can assign custom labels to pipeline runs, such as vertex-ai-pipelines-run-billing-id, and export billing data to BigQuery to analyze resource usage and costs associated with each pipeline run. By correlating these costs with model performance metrics, you can get precise artificial intelligence cost estimation. |
MLflow + Custom ROI Script | experiment tracking custom metrics logging model versioning integration with custom ROI scripts | MLflow itself doesn’t offer a built-in ROI calculator, but it can help calculate ROI using custom scripts. You can log key metrics like model accuracy, cost, and business-specific values using MLflow Tracking, then use a custom Python script to compute ROI based on those metrics. This setup allows you to version and reproduce ROI evaluations over time. |
Amazon SageMaker Studio + Cost Explorer | AWS pricing calculator cost and usage reports (CUR) CloudWatch Metrics SageMaker Model Monitor cost allocation tags | Amazon SageMaker doesn’t include a built-in ROI calculator but provides tools to estimate ROI. By using the AWS Pricing Calculator, you can project costs for various SageMaker services. Combining this with performance metrics from SageMaker Model Monitor and CloudWatch allows you to assess the financial impact of your ML models. |
Microsoft Azure Machine Learning Cost Management | Azure pricing calculator total Cost of Ownership (TCO) calculator Azure cost management and billing budgeting and forecasting tools performance metrics monitoring | Microsoft Azure Machine Learning provides tools to estimate and manage ROI, though it doesn’t offer a dedicated ROI calculator. You can use the Azure Pricing Calculator to estimate costs for various services and the Total Cost of Ownership (TCO) Calculator to compare on-premises and cloud expenses. Additionally, Azure Cost Management and Billing allows you to monitor spending, set budgets, and analyze cost trends. By combining these tools with performance metrics from Azure Machine Learning, you can assess the financial impact of your AI projects. |
Visual ROI Calculator Framework
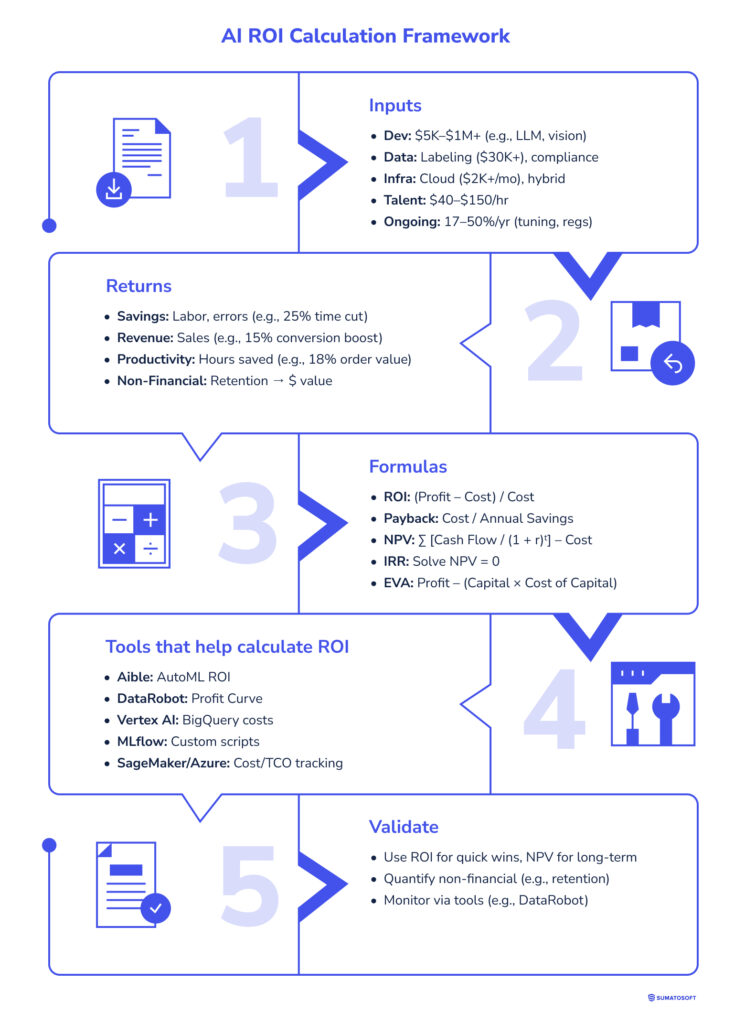
Real-World AI Project Benchmarks
It’s always a good idea to learn by example, so we gathered a list of AI implementation cases of different sizes and complexity. If you need more examples, we encourage you to read this article with 100 cases of AI implementations. Some of the examples were taken from there.
Note: Some numbers in cases are calculated approximations based on the available information. For precise numbers, it’s necessary to contact the appropriate companies.
Case #1: Healthcare – AI-Powered Imaging Analysis
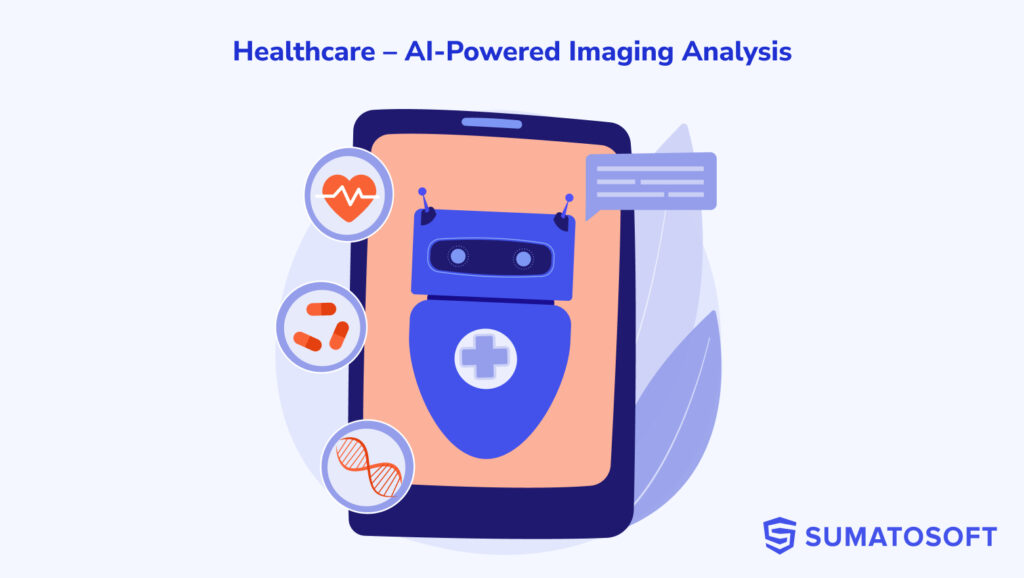
Source: BHM Healthcare Solutions
Investment: $950,000 (software licensing: $500,000; hardware upgrades: $200,000; staff training: $100,000; integration: $150,000).
Duration: 18 months.
Outcome: annual cost savings of $1.2 million, increased revenue of $800,000, and improved patient outcomes valued at $500,000.
Case #2: Banking – Sumitomo Mitsui Banking Corporation (SMBC) with dotData’s AutoML 2.0 Platform
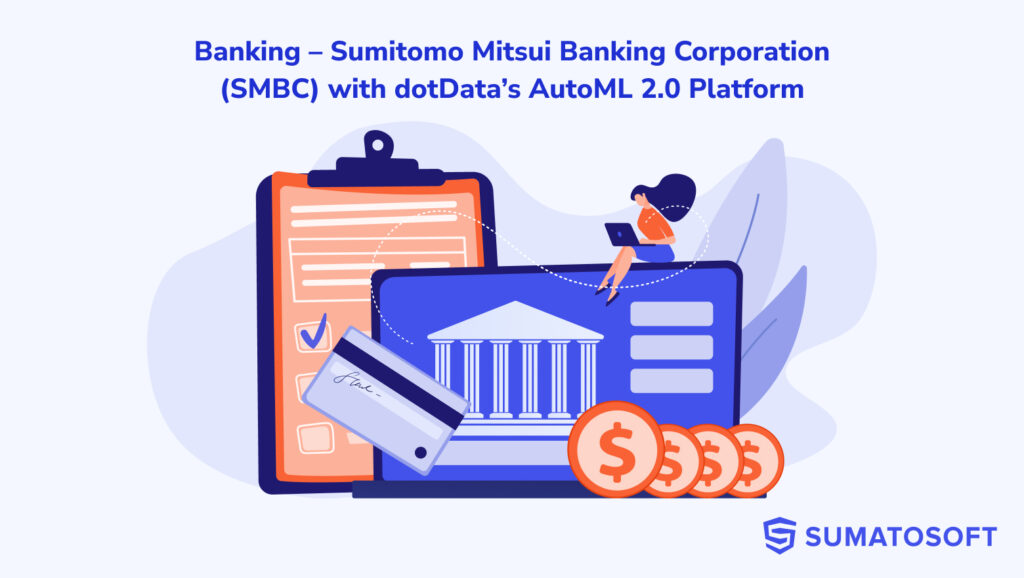
Source: research AIMultiple, “100 AI Use Cases with Real-Life Examples in 2025”
Overview: Sumitomo Mitsui Banking Corporation, a major Japanese bank, implemented dotData’s AutoML 2.0 platform to enhance its AI and machine learning capabilities for data-driven decision-making across various banking operations.
Investment: while exact investment figures for SMBC’s implementation are not publicly disclosed, based on industry benchmarks for enterprise AutoML solutions, we estimated that such a solution would cost large organizations between $500,000 and $2 million annually, including software, cloud, and support costs. SMBC also likely invested in data infrastructure upgrades and training for data scientists, estimated at $200,000–$500,000 for initial setup and onboarding.
Duration: 6–12 months.
Output and Results: accelerated AI model development by 48x, reducing the time to build and deploy models from months to days, developed over 100 AI models per year, compared to a previous capacity of 2–5 models annually with traditional methods, achieved an estimated ROI of over 400% due to increased efficiency, reduced manual labor, and improved business outcomes.
Case #3: Insurance – AXA with Azure OpenAI Service
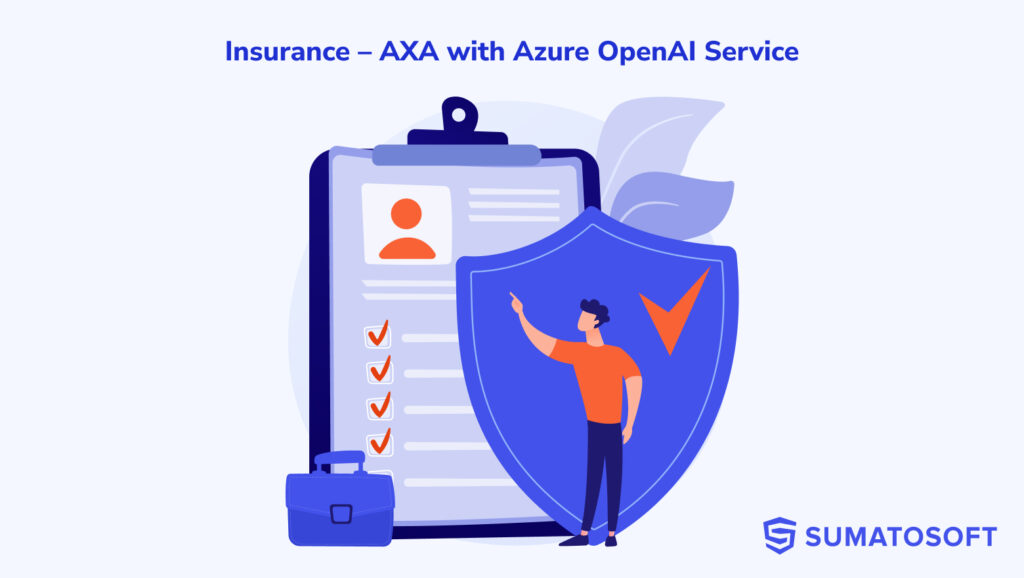
Source: Microsoft Blog, 2025.
Overview: AXA, a leading global insurance company, implemented AXA Secure GPT, a generative AI platform built on Microsoft’s Azure OpenAI Service.
Investment: ~$1M–$3M (estimated for Azure OpenAI licensing, cloud infrastructure, and training).
Duration: 9–12 months.
Output:
- developed AXA Secure GPT, enabling 30,000+ employees to use generative AI safely;
- 25% reduction in time spent on data analysis and report generation;
- 15% increase in employee productivity for administrative tasks;
- ~200% ROI within 18 months;
- enhanced data security and compliance with the proprietary AI platform.
Case $4: Consumer Goods – Coca-Cola with AI-Powered Digital Marketing
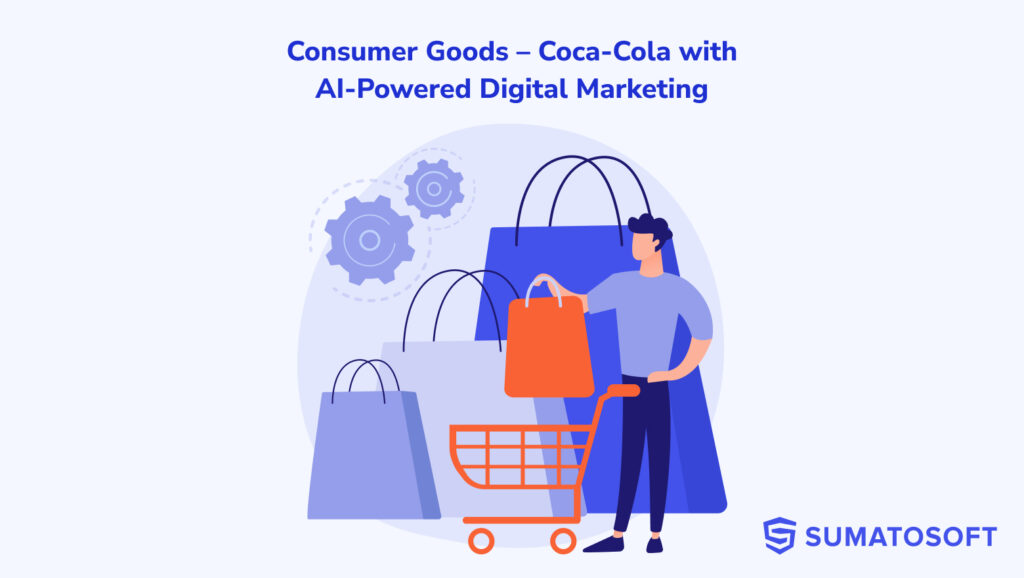
Source: AdWeek, 2024.
Overview: Coca-Cola, a global leader in the beverage and consumer goods industry, leverages the Blackout Platform to deploy AI-powered digital marketing campaigns.
Investment: ~$2M–$5M (platform development, AI tools, and marketing integration).
Duration: 12–18 months.
Output:
- 20% increase in campaign engagement rates;
- 15% boost in sales conversions from targeted ads;
- 30% reduction in ad spend waste through predictive analytics;
- ~300% ROI within 2 years from improved marketing efficiency;
- reached 10M+ personalized customer interactions monthly.
Case #5: E-commerce – Bookshop.org with AI-Powered Recommendation Engine
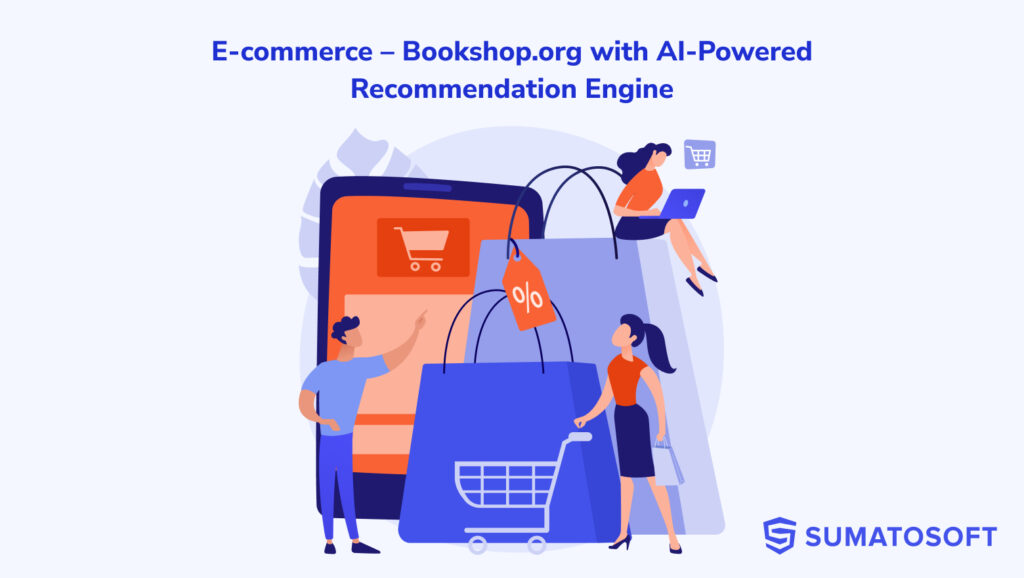
Source: Forbes, 2024
Overview: Bookshop.org, an eCommerce platform for independent book retail, implemented an AI-powered recommendation engine to enhance its online shopping experience.
Investment: ~$100K–$300K.
Duration: 6–9 months.
Output:
- 18% increase in average order value from personalized book suggestions;
- 25% higher customer retention rate due to tailored recommendations;
- 12% boost in conversion rates on product pages;
- ~200% ROI within 1 year from increased sales;
- served 500K+ monthly users with personalized book picks.
Summary: What to Remember
- AI development costs vary widely: $5,000–$50,000 for simple chatbots, $50,000–$150,000 for moderate ML projects, and $150,000–$1M+ for complex LLM or computer vision systems. Key factors defining AI app development costs include development model, AI type, project complexity and goals, data, talent, infrastructure, and third-party APIs or model usage.
- Plan for ongoing costs: allocate 17–30% of initial costs annually for model fine-tuning, infrastructure scaling, AI model monitoring, compliance updates, and post-deployment support.
- Maximize roi: use ROI models (e.g., Payback Period, NPV) to justify investments. Focus on measurable outcomes like cost savings, revenue growth, or productivity gains. Non-financial benefits (e.g., customer satisfaction, data maturity) also add value.
- 5 evaluation metrics for AI development: payback period, ROI, NPV, IRR, and EVA help estimate profitability, assess long-term value, and compare project options. Combine these with non-financial metrics like speed-to-market, employee productivity, customer experience, and brand visibility to see a complete picture.
- Use software tools for ROI calculation: several platforms offer tools to calculate AI app development costs and estimate financial impact, even without a built-in ROI calculator. Solutions like Aible and DataRobot provide guided ROI optimization and custom evaluation metrics, while platforms such as Google Vertex AI, MLflow, Amazon SageMaker, and Microsoft Azure allow cost tracking, performance monitoring, and integration with financial analysis tools.
- Real-world benchmarks: cases like AXA (~$1M–$3M, 200% ROI) and Bookshop.org ($100K–$300K, 200% ROI) show AI’s potential for significant returns when planned effectively.
- Next steps: if you are planning an AI development or want to estimate the AI development costs of your project – contact us.
Let’s start
If you have any questions, email us [email protected]
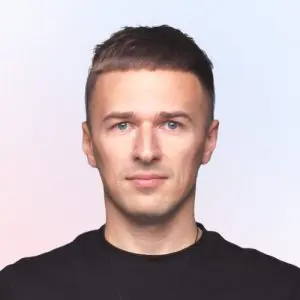